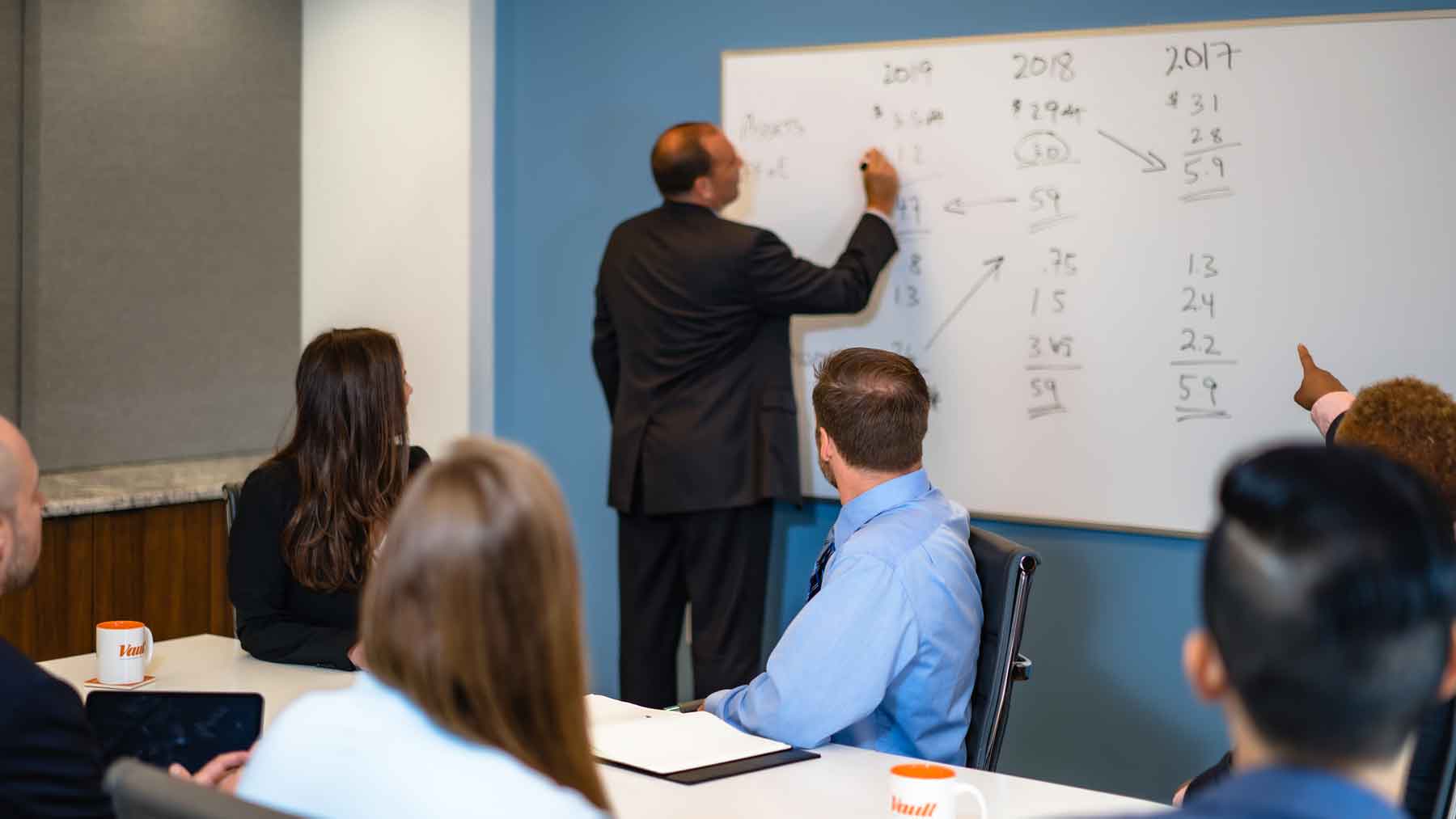
Every April, people around the world celebrate MATHEMATICS AND STATISTICS AWARENESS MONTH (MASAM). This effort began in 1986, when President Ronald Reagan declared one week in April as Mathematics Awareness Week. As popularity grew and more people turned to statistics-based jobs, the original week became a month and statistics was added to the title.
Statistics makes it possible to understand and interpret data. By using statistical methods and knowledge, users can collect, analyze, and present results on a wide range of topics. Most commonly used to make predictions and discoveries, statistics are important to every business in every field. When interpreted right, statistics help leaders make informed decisions. Unfortunately, statistics are easily misinterpreted, leading to inaccurate results and poor conclusions.
For MASAM, Vault Consulting provides the following information to help you better understand this complex subject.
1. Correlation: When I move, you move.
Two variables are correlated if there is a relationship between their movements. If Variable A generally goes up when B goes up, they are positively correlated. If Variable A generally goes up when B goes down, they are negatively correlated. Critically, correlation is all about the change in a variable, not the actual level of a variable.
Correlation does NOT mean variable A can substitute for variable B (i.e. that they are the same thing). For example, let’s say you are buying a Chipotle Burrito, and it costs $10. You can either pay for the Burrito with 10 one-dollar bills, or 1,000 pennies. The price of the Burrito in terms of one-dollar bills (10 bills) and pennies (1,000 pennies), is perfectly correlated. If the burrito price doubles to $20, then the amount of one-dollar bills and pennies you would need to buy the burrito both double. Obviously, however, pennies aren’t a 1 to 1 substitute for dollar bills. Otherwise, you would be eager to trade a one-dollar bill for 2 pennies.
2. Causation: You move because I move.
Correlation doesn’t necessarily mean causation. However, a more complete catch phrase would be that “Correlation means causation, if and only if the correlation still holds when all other relevant variables are held constant”
In other words, the statement “A causes B” really means that an increase in A will increase B in a world where no other variables change. For example, it is likely that a city’s number of police officers per capita is positively correlated with the crime rate (i.e. that as the number of police increase in a city, crime also increases). Does this mean that police officers are causing crime? Of course not. Cities with higher crime rates are enlisting more police than areas with lower crime. To answer the question about causation, you need to observe what the effect of an increase in police officers has on crime, holding the city, the period of time, and all other relevant factors constant.
3. Statistical Significance: Sometimes Significance isn’t that Significant.
Most people have encountered the term “Statistically Significant”, but far fewer really know what it means. In layman’s terms, statistically significance means that an observed effect or difference is unlikely to be the result of sample error (more on this later). It does NOT, mean that the observed effect is necessarily important.
So what is statistical significance? To determine statistical significance, you need to begin with some assumptions about a distribution. A distribution is a curve that shows how frequently data occurs at different points of a variable. The higher the curve, the higher the frequency at that point on the curve.
Whenever you take a sample of a population, you never get a perfect representation of that population. Randomness and noise will ensure that the average characteristics of that sample will not exactly equal the population average. As the number of observations in the sample increases, the sample becomes less noisy, and you become more confident that your sample is representative of the underlying population.
For example, suppose that you want to determine whether the height difference between American men and American women is statistically significant. To do this, you gather 2 samples: 1 sample is the heights of 1,000 American males, and 1 sample is the heights of 1,000 American females. Let’s say that the average height of males in the sample is 5’9” while the average height of females in the sample is 5’ 6”. If this difference is statistically significant, you are saying that the likelihood that you would observe the 3-inch height difference given that American Males and American Females are the same height is small (usually 1- 5% is the threshold used). This statement does not indicate that the 3-inch difference is important, just that the two populations are statistically non-identical. As the size of your samples increase, smaller differences will become statistically significant. However, the question of whether the differences matter will depend on a broader context. Accordingly, when presenting research or discussing policy, it’s not enough to know whether an observed difference is statistically significant, you must also ask whether it is statistically important.
4. Statistical Noise: The rule of small numbers.
Item 3 discussed Statistical significance. A key piece of that lesson is that large sample sizes are less affected by random noise. Conversely, small samples are noisy. This means you are more likely to observe outliers in small samples than in big samples. This fact has important implications for what can be called the rule of small numbers.
To motivate this idea, imagine that you are the Secretary of the Department of Education. You are reading a report on test scores and school sizes. In the first paragraph you read the following result:
“Of the top 10% of high schools based on math test scores, 90% had fewer than 600 students in the school.”
Like many people, you might immediately jump to a causal connection between school size and test scores. You might conclude that small schools create a better education environment and that reducing school sizes will result in better test scores. Now imagine that you read the following result in the next paragraph.
“Of the bottom 10% of high schools based on math test scores, 90% had fewer than 600 students in the school.”
Results 1 and 2 seem to contradict each other, but they are both consistent with the rule of small numbers. Smaller samples are more likely to yield outliers. Some small schools might have a lot of high test scoring kids, others will have more low test scoring kids. Thus, whenever you read a statistical statement comparing two groups, be sure to think about whether the rule of small numbers may be impacting the results.
5. Regression to the Mean: Impress your friends with easy predictions.
Regression to the mean is a critical concept needed to make predictions, but many people ignore it entirely. Childhood height is a good example that illustrates how failure to account for regression to the mean can result in poor predictions.
As an example, suppose that your friend’s son Bill is 4’8”. This places Bill in the 99.5th percentile of the height distribution for his age group with the average 8-year-old American male being 4’ 2” tall. If Bill stays in the 99.5th percentile, he will grow to be 6’ 5”. Knowing this, many people will predict that Bill will on average will grow to about 6’5”, give or take a few inches. However, the correct prediction is that Bill will be shorter than 6’5” as an adult. This is because, his height, on average should move closer to the 50th percentile as he develops. This is known as regression to the mean.
If the correlation between childhood and adult height is 50%, then a rough prediction for the Bill’s adult height will be 6’1”. This height is halfway between the average adult male’s height (5’9”) and the naïve prediction where Bill stays in the same percentile (6’5”).
Regression to the mean underlies many phenomena. Professional athletes who sign contracts after a career year, tend to underperform their contract in subsequent years. Children of very tall parents tend to be shorter than their parents. Stocks which perform very strongly in one year, tend to underwhelm in subsequent years. In many cases, when a person or phenomenon deviates far from the mean in one direction or the other, a simple and easy prediction is that it will move closer to the average.
6. Simpson’s Paradox : Don’t always trust the total.
Imagine you are a kidney surgeon and are reviewing the efficacy of kidney stone treatments.
You have two primary choices:
Treatment A) open surgery on the kidney
Treatment B) making a small incision through the kidney and removing the stones.
According to a peer reviewed study which compared the two kidney stone operations over a sample of 350 surgeries each, treatment A has a success rate of 78% while treatment B has a success rate of 83%.
Treatment A (273/350 surgeries were successful)
Treatment B (289/350 surgeries were successful)
Clearly treatment B is more effective, right? Actually, this isn’t the full story. The above statistic doesn’t break out the surgeries by the situation. It turns out that treatment A was used primarily for kidney surgeons for large stones, whereas treatment B was used primarily for small stones. Because operations to treat small stones have a higher success rate, treatment B’s success rate was biased upwards.
7. Bayesian Updating: No one thinks like this.
Justin is 6’ 9. He has a 40” vertical and was the star of his high school basketball team. He was below average at math in high school.
Is Justin more likely to be:
- An NBA Basketball Player
- A Certified Public Accountant
If you immediately reacted by choosing A, then like many of us, you are not taking into account your Bayesian Priors (i.e. what is the base likelihood that someone is in the NBA vs is an accountant). While Justin’s description itself is more representative of an NBA basketball player, one needs to account for the fact that there are over 660,000 CPAs in the USA but only about 660 players in the NBA. That means, if you were to pick someone at random, they would be 1000X more likely to be a CPA than an NBA basketball player!
This initial probability/likelihood of being an NBA player vs an accountant is called a Bayesian Prior. To update Justin’s probability of being an NBA Basketball player vs. a CPA, you need to do the following calculation:
New Likelihood = Prior Likelihood * Update Factor
Let’s say that Justin’s description makes him 100 times more likely to play in the NBA. Then his update factor is 100. So given a prior likelihood of 1/1000 and an update factor of 100, what are the new odds that Justin is an NBA player vs. being a CPA?
New Likelihood = (1/1000) * 100 = 1/10
So even though Justin’s description increases the odds that he is in the NBA, he is still 10 times more likely to be a CPA than he is an NBA basketball player. This is because there are so many more CPAs than NBA players. In summary always remember your Bayesian priors.
Vault Consulting provides outsourced accounting and market research for nonprofits, associations, and their affiliates. Please contact us for more information about collecting and analyzing market data.